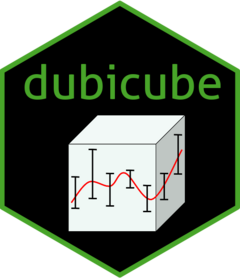
Perform bootstrapping over a data cube for a calculated statistic
Source:R/bootstrap_cube.R
bootstrap_cube.Rd
This function generate samples
bootstrap replicates of a statistic applied
to a data cube. It resamples the data cube and computes a statistic fun
for
each bootstrap replicate, optionally comparing the results to a reference
group (ref_group
).
Usage
bootstrap_cube(
data_cube,
fun,
...,
grouping_var,
samples = 1000,
ref_group = NA,
seed = NA,
progress = FALSE
)
Arguments
- data_cube
A data cube object (class 'processed_cube' or 'sim_cube', see
b3gbi::process_cube()
) or a dataframe (from$data
slot of 'processed_cube' or 'sim_cube'). To limit runtime, we recommend using a dataframe with custom function asfun
.- fun
A function which, when applied to
data_cube
returns the statistic(s) of interest. This function must return a dataframe with a columndiversity_val
containing the statistic of interest.- ...
Additional arguments passed on to
fun
.- grouping_var
A string specifying the grouping variable(s) for the bootstrap analysis. The output of
fun(data_cube)
returns a row per group.- samples
The number of bootstrap replicates. A single positive integer. Default is 1000.
- ref_group
A string indicating the reference group to compare the statistic with. Default is
NA
, meaning no reference group is used.- seed
A positive numeric value setting the seed for random number generation to ensure reproducibility. If
NA
(default), thenset.seed()
is not called at all. If notNA
, then the random number generator state is reset (to the state before calling this function) upon exiting this function.- progress
Logical. Whether to show a progress bar. Set to
TRUE
to display a progress bar,FALSE
(default) to suppress it.
Value
A dataframe containing the bootstrap results with the following columns:
sample
: Sample ID of the bootstrap replicateest_original
: The statistic based on the full dataset per grouprep_boot
: The statistic based on a bootstrapped dataset (bootstrap replicate)est_boot
: The bootstrap estimate (mean of bootstrap replicates per group)se_boot
: The standard error of the bootstrap estimate (standard deviation of the bootstrap replicates per group)bias_boot
: The bias of the bootstrap estimate per group
Details
Bootstrapping is a statistical technique used to estimate the distribution of a statistic by resampling with replacement from the original data (Davison & Hinkley, 1997; Efron & Tibshirani, 1994). In the case of data cubes, each row is sampled with replacement. Below are the common notations used in bootstrapping:
Original Sample Data: \(\mathbf{X} = \{X_1, X_2, \ldots, X_n\}\)
The initial set of observed data points. Here, \(n\) is the sample size. This corresponds to the number of cells in a data cube or the number of rows in tabular format.
Statistic of Interest: \(\theta\)
The parameter or statistic being estimated, such as the mean \(\bar{X}\), variance \(\sigma^2\), or a biodiversity indicator. Let \(\hat{\theta}\) denote the estimated value of \(\theta\) calculated from the complete dataset \(\mathbf{X}\).
Bootstrap Sample: \(\mathbf{X}^* = \{X_1^*, X_2^*, \ldots, X_n^*\}\)
A sample of size \(n\) drawn with replacement from the original sample \(\mathbf{X}\). Each \(X_i^*\) is drawn independently from \(\mathbf{X}\).
A total of \(B\) bootstrap samples are drawn from the original data. Common choices for \(B\) are 1000 or 10,000 to ensure a good approximation of the distribution of the bootstrap replications (see further).
Bootstrap Replication: \(\hat{\theta}^*_b\)
The value of the statistic of interest calculated from the \(b\)-th bootstrap sample \(\mathbf{X}^*_b\). For example, if \(\theta\) is the sample mean, \(\hat{\theta}^*_b = \bar{X}^*_b\).
Bootstrap Statistics:
Bootstrap Estimate of the Statistic: \(\hat{\theta}_{\text{boot}}\)
The average of the bootstrap replications:
$$\hat{\theta}_{\text{boot}} = \frac{1}{B} \sum_{b=1}^B \hat{\theta}^*_b$$
Bootstrap Bias: \(\text{Bias}_{\text{boot}}\)
This bias indicates how much the bootstrap estimate deviates from the original sample estimate. It is calculated as the difference between the average bootstrap estimate and the original estimate:
$$\text{Bias}_{\text{boot}} = \frac{1}{B} \sum_{b=1}^B (\hat{\theta}^*_b - \hat{\theta}) = \hat{\theta}_{\text{boot}} - \hat{\theta}$$
Bootstrap Standard Error: \(\text{SE}_{\text{boot}}\)
The standard deviation of the bootstrap replications, which estimates the variability of the statistic.
References
Davison, A. C., & Hinkley, D. V. (1997). Bootstrap Methods and their Application (1st ed.). Cambridge University Press. doi:10.1017/CBO9780511802843
Efron, B., & Tibshirani, R. J. (1994). An Introduction to the Bootstrap (1st ed.). Chapman and Hall/CRC. doi:10.1201/9780429246593
See also
Other uncertainty:
add_effect_classification()
,
calculate_bootstrap_ci()
Examples
# Get example data
# install.packages("remotes")
# remotes::install_github("b-cubed-eu/b3gbi")
library(b3gbi)
cube_path <- system.file(
"extdata", "denmark_mammals_cube_eqdgc.csv",
package = "b3gbi")
denmark_cube <- process_cube(
cube_path,
first_year = 2014,
last_year = 2020)
# Function to calculate statistic of interest
# Mean observations per year
mean_obs <- function(data) {
out_df <- aggregate(obs ~ year, data, mean) # Calculate mean obs per year
names(out_df) <- c("year", "diversity_val") # Rename columns
return(out_df)
}
mean_obs(denmark_cube$data)
#> year diversity_val
#> 1 2014 11.553740
#> 2 2015 11.532206
#> 3 2016 5.532491
#> 4 2017 5.703888
#> 5 2018 5.598413
#> 6 2019 4.802676
#> 7 2020 4.972163
# Perform bootstrapping
# \donttest{
bootstrap_mean_obs <- bootstrap_cube(
data_cube = denmark_cube$data,
fun = mean_obs,
grouping_var = "year",
samples = 1000,
seed = 123,
progress = FALSE)
head(bootstrap_mean_obs)
#> sample year est_original rep_boot est_boot se_boot bias_boot
#> 1 1 2014 11.55374 11.08362 11.55352 1.484546 -0.0002192649
#> 2 2 2014 11.55374 12.50984 11.55352 1.484546 -0.0002192649
#> 3 3 2014 11.55374 11.00085 11.55352 1.484546 -0.0002192649
#> 4 4 2014 11.55374 11.62364 11.55352 1.484546 -0.0002192649
#> 5 5 2014 11.55374 13.37887 11.55352 1.484546 -0.0002192649
#> 6 6 2014 11.55374 11.70199 11.55352 1.484546 -0.0002192649
# }