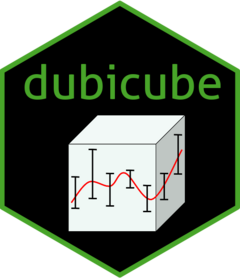
Leave-one-out cross-validation for data cubes
Source:R/cross_validate_cube.R
cross_validate_cube.Rd
This function performs leave-one-out (LOO) or k-fold (experimental) cross-validation (CV) on a biodiversity data cube to assess the performance of a specified indicator function. It partitions the data by a specified variable, calculates the specified indicator on training data, and compares it with the true values to evaluate the influence of one or more categories on the final result.
Arguments
- data_cube
A data cube object (class 'processed_cube' or 'sim_cube', see
b3gbi::process_cube()
) or a dataframe (cf.$data
slot of 'processed_cube' or 'sim_cube'). Ifprocessed_cube = TRUE
(default), this must be a processed or simulated data cube that contains a$data
element.- fun
A function which, when applied to
data_cube$data
returns the statistic(s) of interest (or justdata_cube
in case of a dataframe). This function must return a dataframe with a columndiversity_val
containing the statistic of interest.- ...
Additional arguments passed on to
fun
.- grouping_var
A character vector specifying the grouping variable(s) for
fun
. The output offun(data_cube)
returns a row per group.- out_var
A string specifying the column by which the data should be left out iteratively. Default is
"taxonKey"
which can be used for leave-one-species-out CV.- crossv_method
Method of data partitioning. If
crossv_method = "loo"
(default),S = number of unique values in out_var
training partitions are created containingS - 1
rows each. Ifcrossv_method = "kfold"
, the aggregated data is split the data intok
exclusive partitions containingS / k
rows each. K-fold CV is experimental and results should be interpreted with caution.- k
Number of folds (an integer). Used only if
crossv_method = "kfold"
. Default 5.- max_out_cats
An integer specifying the maximum number of unique categories in
out_var
to leave out iteratively. Default is1000
. This can be increased if needed, but keep in mind that a high number of categories inout_var
may significantly increase runtime.- processed_cube
Logical. If
TRUE
(default), the function expectsdata_cube
to be a data cube object with a$data
slot. IfFALSE
, the function expectsdata_cube
to be a dataframe.- progress
Logical. Whether to show a progress bar. Set to
TRUE
to display a progress bar,FALSE
(default) to suppress it.
Value
A dataframe containing the cross-validation results with the following columns:
Cross-Validation id (
id_cv
)The grouping variable
grouping_var
(e.g., year)The category left out during each cross-validation iteration (specified
out_var
with suffix '_out' in lower case)The computed statistic values for both training (
rep_cv
) and true datasets (est_original
)Error metrics: error (
error
), squared error (sq_error
), absolute difference (abs_error
), relative difference (rel_error
), and percent difference (perc_error
)Error metrics summarised by
grouping_var
: mean relative difference (mre
), mean squared error (mse
) and root mean squared error (rmse
)
See Details section on how these error metrics are calculated.
Details
This function assesses the influence of each category in out_var
on the
indicator value by iteratively leaving out one category at a time, similar to
leave-one-out cross-validation. K-fold CV works in a similar fashion but is
experimental and will not be covered here.
Original Sample Data: \(\mathbf{X} = \{X_{11}, X_{12}, X_{13}, \ldots, X_{sn}\}\)
The initial set of data points, where there are \(s\) different categories in
out_var
and \(n\) total samples across all categories (= the sample size). \(n\) corresponds to the number of cells in a data cube or the number of rows in tabular format.
Statistic of Interest: \(\theta\)
The parameter or statistic being estimated, such as the mean \(\bar{X}\), variance \(\sigma^2\), or a biodiversity indicator. Let \(\hat{\theta}\) denote the estimated value of \(\theta\) calculated from the complete dataset \(\mathbf{X}\).
Cross-Validation (CV) Sample: \(\mathbf{X}_{-s_j}\)
The full dataset \(\mathbf{X}\) excluding all samples belonging to category \(j\). This subset is used to investigate the influence of category \(j\) on the estimated statistic \(\hat{\theta}\).
CV Estimate for Category \(\mathbf{j}\): \(\hat{\theta}_{-s_j}\)
The value of the statistic of interest calculated from \(\mathbf{X}_{-s_j}\), which excludes category \(j\). For example, if \(\theta\) is the sample mean, \(\hat{\theta}_{-s_j} = \bar{X}_{-s_j}\).
Error Measures:
The Error is the difference between the statistic estimated without category \(j\) (\(\hat{\theta}_{-s_j}\)) and the statistic calculated on the complete dataset (\(\hat{\theta}\)).
$$\text{Error}_{s_j} = \hat{\theta}_{-s_j} - \hat{\theta}$$
The Relative Error is the absolute error, normalised by the true estimate \(\hat{\theta}\) and a small error term \(\epsilon = 10^{-8}\) to avoid division by zero.
$$\text{Rel. Error}_{s_j} = \frac{|\hat{\theta}_{-s_j} - \hat{\theta}|}{\hat{\theta} +\epsilon}$$
The Percent Error is the relative error expressed as a percentage.
$$\text{Perc. Error}_{s_j} = \text{Rel. Error}_{s_j} \times 100 \%$$
Summary Measures:
The Mean Relative Error (MRE) is the average of the relative errors over all categories.
$$\text{MRE} = \frac{1}{s} \sum_{j=1}^s \text{Rel. Error}_{s_j}$$
The Mean Squared Error (MSE) is the average of the squared errors.
$$\text{MSE} = \frac{1}{s} \sum_{j=1}^s (\text{Error}_{s_j})^2$$
The Root Mean Squared Error (RMSE) is the square root of the MSE.
$$\text{RMSE} = \sqrt{\text{MSE}}$$
Examples
if (FALSE) { # \dontrun{
# After processing a data cube with b3gbi::process_cube()
# Function to calculate statistic of interest
# Mean observations per year
mean_obs <- function(data) {
out_df <- aggregate(obs ~ year, data, mean) # Calculate mean obs per year
names(out_df) <- c("year", "diversity_val") # Rename columns
return(out_df)
}
mean_obs(processed_cube$data)
# Perform leave-one-species-out CV
cv_mean_obs <- cross_validate_cube(
data_cube = processed_cube,
fun = mean_obs,
grouping_var = "year",
out_var = "taxonKey",
crossv_method = "loo",
progress = FALSE
)
head(cv_mean_obs)
} # }